What are the best Github Repos for ControlNet?
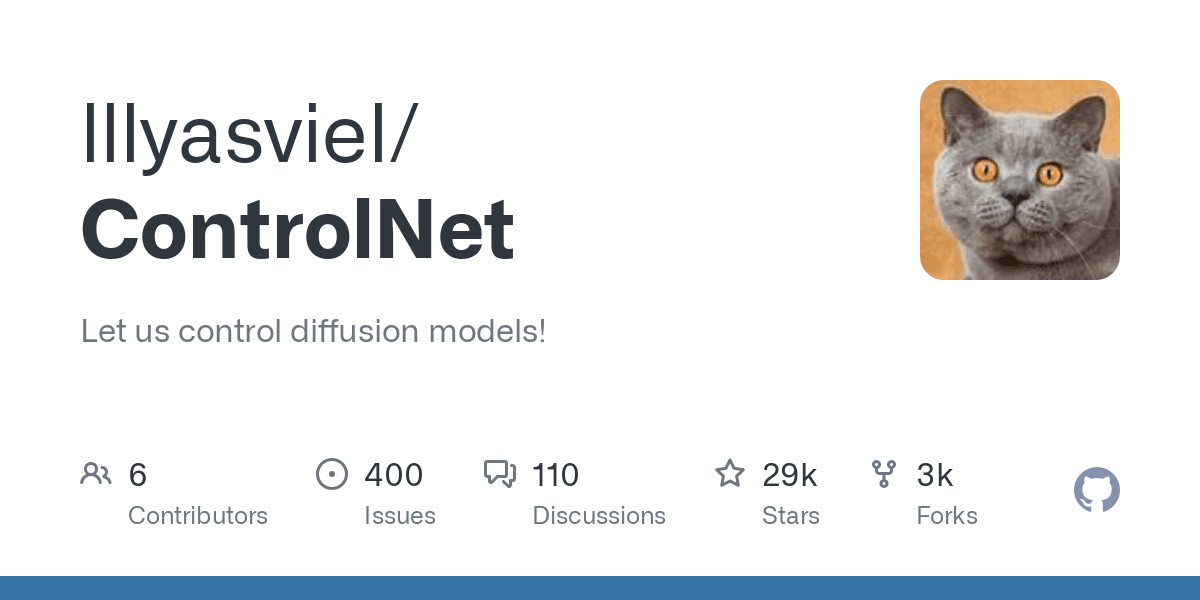
Challenges and Limitations of IP Adapters: Navigating the Frontiers of AI Image Generation
IP Adapters have revolutionized AI image generation, with variants like IPAdapter FaceID and IP Adapter ControlNet pushing the boundaries of what's possible. However, as with any cutting-edge technology, these tools come with their own set of challenges and limitations. Let's explore some of the key issues facing IP Adapters in today's landscape.
Generalization and Data Dependency
One of the primary challenges for IP Adapters, including IPAdapter FaceID, is the issue of generalization. The effectiveness of these models can vary significantly depending on the diversity and quality of their training data1. This limitation becomes particularly apparent when dealing with faces or subjects that differ substantially from the training set, potentially leading to inconsistent results or reduced quality in generated images.
Balancing Influence and Control
For users of IP Adapter ControlNet and similar tools, finding the right balance between the influence of the image prompt and text instructions can be a delicate process2. This challenge is especially pronounced when attempting to generate complex scenes or when trying to maintain specific stylistic elements while altering other aspects of the image.
Integration and Compatibility
While platforms like ComfyUI have made great strides in integrating IP Adapters, including IPAdapter FaceID and ControlNet variants, compatibility issues can still arise3. Users may encounter challenges when trying to combine different models or when integrating IP Adapters with other AI image generation tools, potentially limiting the full potential of these technologies.
Ethical and Copyright Concerns
As with many AI-driven content creation tools, IP Adapters face ongoing discussions about the ethical use of reference images and potential copyright implications1. This is particularly relevant for IPAdapter FaceID, where the use of personal likenesses raises questions about consent and image rights.
Performance and Resource Requirements
High-quality results from IP Adapters, especially when combining multiple models like IPAdapter FaceID with ControlNet, can be computationally intensive. This may limit accessibility for users without access to powerful hardware, potentially creating a barrier to entry for some creators4.
Overcoming Limitations in Training
Recent discussions in the developer community highlight challenges in training IP Adapters, particularly for specialized applications like face recognition. Issues such as overfitting on small datasets and the need for large, diverse training sets have been noted as significant hurdles2.
Conclusion
While IP Adapters, including IPAdapter FaceID and IP Adapter ControlNet, offer powerful capabilities for AI image generation, they are not without their challenges. As the technology continues to evolve, addressing these limitations will be crucial for wider adoption and more consistent results. Researchers and developers are actively working on solutions, from improved training methodologies to more efficient integration with platforms like ComfyUI IPAdapter plus.
As users and creators, staying informed about these challenges can help set realistic expectations and guide the effective use of these powerful tools in various creative and professional applications.
Citations: